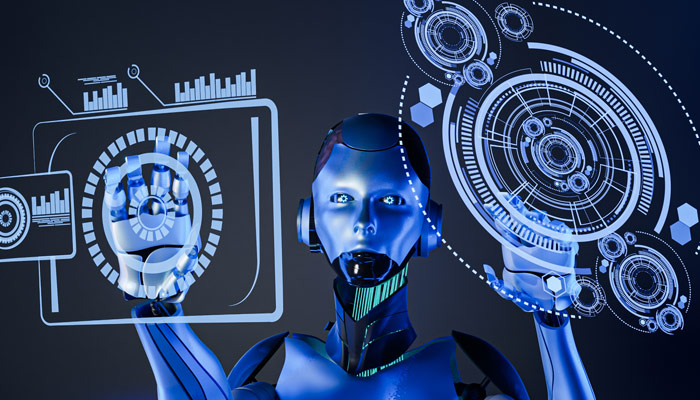
Regulating the Robots
Review of “FDA Commissioner Robert Califf on Setting Guardrails for AI in Health Care”
Original conversation and article appeared in JAMA Review, by Roy Perlis, MD, MSc, and Jennifer Abbasi
In a timely interview with JAMA, Robert Califf, MD—Commissioner of the U.S. Food and Drug Administration (FDA)—discusses concerns regarding the approval and integration of artificial intelligence (AI) in healthcare. One of his primary concerns is how to reliably and dynamically ensure AI systems are safe and effective for patient care. He emphasizes the need for robust data to validate AI algorithms, given the high stakes involved in healthcare decisions.
Dr. Califf also highlights the importance of transparency in AI systems, ensuring that healthcare providers and patients understand how AI-derived decisions are made. He stresses the responsibility the FDA has to provide a regulatory framework to keep pace with rapid technological advancements while maintaining public trust in those innovations.
The Black Box Problem
We sometimes forget how much of the healthcare industry is based on blind trust. Trust in our doctor, in our technology and our drug industry. That trust is based on the ability to track, verify and measure an innovation’s impact on health outcomes. Of all challenges that regulators face in approving AI based diagnostic solutions the single greatest is the concept of accountability.
Black box AI refers to artificial intelligence systems whose internal processes and decision-making mechanisms are hidden or not understandable to users. These systems produce outcomes without providing insights into how the results were reached, making them opaque and impenetrable.
Robert Califf, MD: Key Points on Handling the “Black Box Problem”
- Continuous Monitoring: AI algorithms require ongoing oversight similar to monitoring an ICU patient due to their evolving nature.
- Regulatory Safeguards: Implementing safety mechanisms to ensure AI systems do not make inaccurate predictions and remain effective over time.
- Collaborative Responsibility: Encouraging health systems and professional societies to engage in self-regulation alongside the FDA.
- Transparency in Performance: Health systems must track AI’s sensitivity, specificity, and overall accuracy continuously.
- Legal Authority: Seeking additional legal powers from Congress to strengthen the FDA’s regulatory capabilities for AI technologies.
Large data quantitative models use a plethora of variables when establishing a decision rule. Not all of those variables are known at the outset, rather they are determined as the algorithm is trained. A trading algorithm or supply chain management system need not provide a forensic accounting and analysis of exactly “WHY” a decision was made—it’s enough to know that it was and that over time the right decisions will be made on average.
But if someone dies as a result of a machine-driven decision you can be sure family members, regulators and lawyers will want to know why (an identical issue is confounding the adoption of self-driving cars). To address the anticipated difficulties in 2021 the FDA promoted 10 guiding principles for good machine learning practice (GMLP) and have been updating them regularly since.
A Fertile Area Ripe for Innovation
We are still early days but the increased transparency seems to be yielding results as the approval rates of AI/ML enabled devices continue to climb. Over the past decade the FDA has seen a significant increase in the approval of medical devices incorporating Artificial Intelligence (AI) and Machine Learning (ML) technologies. The number of AI/ML-enabled medical devices approved by the FDA each year from 2015 to 2024.
FDA Approvals of AI Medical Devices Surge
FDA authorizations of AI/ML-powered medical devices reached a record high of 221 in 2023
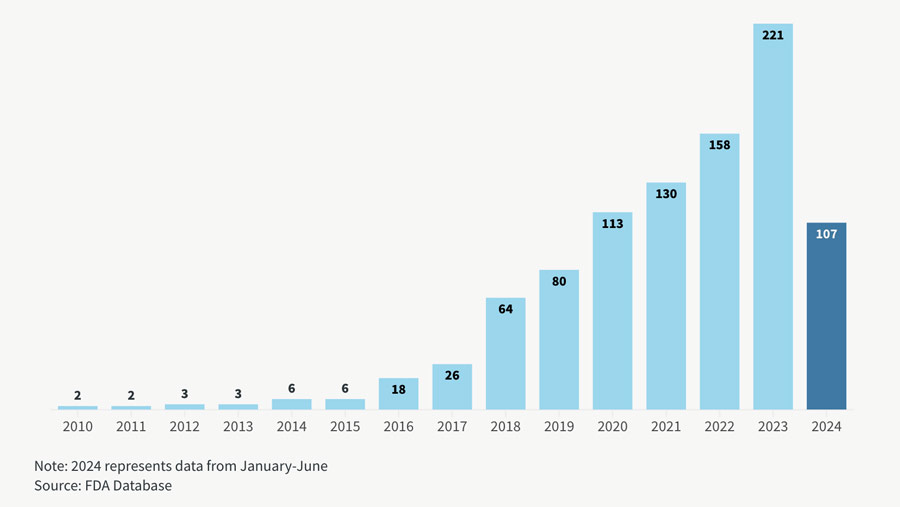
Chart courtesy of Goodwin Law; data through mid-2024.
The trend is the right way but these devices still represent a fraction of the overall total. Currently the lion’s share of approvals are for radiology, comprising more than 75% of the total. But AI products themselves represent less than 40% in that highly penetrated field. While the Theranos debacle could have set the field of automated blood testing back a generation, the pandemic created a need for fast turnaround times and the increased investment attention is beginning to yield results across a wide range of automated diagnostic testing equipment.
Much of those inventions rely on ML/AI solutions and investors should be searching for those opportunities as they are finally about to receive their time in the sun. For example Prenosis just received authorization for the first AI diagnostic test for sepsis. It helps doctors to not only diagnose infections but also predict which patients are most likely to decline in the next 24 hours. The test is built on an AI algorithm that scans 22 different health metrics to come up with a sepsis risk score. And in 2024, Guardant Health’s Shield test received FDA approval as a blood-based screening tool for colon cancer. This test detects circulating tumor DNA (ctDNA) in blood samples, enabling early cancer detection.
By underscoring the FDA’s proactive stance on regulating AI in healthcare, Commissioner Dr. Robert Califf emphasizes the need for a balanced approach that fosters innovation while ensuring safety and efficacy. The FDA’s approval of nearly 1,000 AI-based devices is presented to counter the narrative that AI has yet to be integrated into healthcare. He acknowledges the challenges posed by AI in potentially exacerbating healthcare disparities and calls for collaborative responsibility among health systems, professional societies and the FDA to ensure AI is used intelligently and equitably. Dr. Califf concludes with a hopeful outlook on AI’s potential to improve health outcomes—provided there is mutual accountability and a commitment to transparency in its application.
“We are asking Congress for more legal authority to help make this happen. And I’m very worried about it because I think the main use of AI algorithms today in our health systems is to optimize finances… Our accountability is really for safety and effectiveness… We have to work (within the) ecosystem and persuade.”
~Dr. Robert Califf
Further Reading
No Black Box Analytics: “Explainable AI” Is the gGoal
The U.S. Food and Drug Administration (FDA) has expressed concerns about “black box” diagnostics—AI-driven systems that provide outputs without transparent reasoning. This opacity challenges the FDA’s mandate to ensure safety and efficacy in medical devices. In a presentation titled “Explainable AI and Regulation in Medical Devices,” the FDA emphasized the need for explainable AI, highlighting that while AI can outperform rule-based algorithms, its non-explainable nature poses regulatory challenges.
Without Validation it Is Difficult to Justify Adoption
A study published in Nature Medicine found that more than 40% of AI-enabled medical devices authorized by the FDA between 1995 and 2022 were missing clinical validation data. This lack of transparency and validation poses challenges for regulatory oversight and public trust.
Providing Transparency and Validation Is Necessary
Companies that provide transparent methodologies and robust clinical validation have successfully gained FDA approval. For instance, Prenosis received FDA authorization for its Sepsis Immunoscore, an AI-based diagnostic tool for sepsis. The system analyzes multiple health metrics to generate a sepsis risk score, aiding in early detection and treatment. Prenosis’ commitment to validation and transparency facilitated its approval.
Even Robots Can Get Sloppy
The Advanced Research Projects Agency for Health (ARPA-H) has launched the PRECISE-AI program to tackle a growing concern in healthcare: the decline in accuracy of AI-enabled medical tools over time. This degradation happens as real-world conditions—like patient demographics, clinical practices, and data collection methods—change, making AI systems less effective than when they were first developed. By focusing on tools that continuously monitor and adjust AI performance, the program aims to prevent misdiagnoses and protect patient safety, ensuring these technologies remain reliable as healthcare evolves.